Release time: 2020-07-10 12:21
AI May Help Predict How Depression Symptoms Respond to Treatment
Researchers tested an algorithm for predicting antidepressant effects.
Imagine mental health care professionals having the ability to reliably predict how a person suffering from depression will respond to certain antidepressant medication based on symptoms and brain wave data, rather than trial and error. Recently, researchers developed an artificial intelligence (AI) algorithm that may accelerate precision medicine for depression.
In a new study published on June 22 in JAMA Network Open, researchers used machine learning with pretreatment symptom scores and brain wave data to predict which depressive symptoms would improve with antidepressants.
Major depressive disorder (MDD) is a common mental health condition, affecting an estimated 16 million adults in the United States each year. Fortunately, depression can be treated; unfortunately, however, depression is deeply variegated, with a myriad of possible combinations of symptoms, making pinpointing the right course of treatment a challenge. The frequently used Hamilton Rating Scale for Depression (HRSD) has 21 categories of symptoms, including depressed mood, self-critical, suicidal thoughts, trouble sleeping, agitation, worrying, physical anxiety, appetite changes, and energy loss. This prognostic Stanford study directly addresses this challenge.
Data were collected across 20 sites in five countries from 518 outpatients diagnosed with depression, who were randomized to eight weeks of treatment from one of three different medications: escitalopram oxalate, sertraline hydrochloride, or extended-release venlafaxine hydrochloride.
The team developed a machine learning model called the ElecTreeScore that uses gradient boosting decision trees (GBDTs). They used to model to predict the treatment outcome of antidepressant drugs for each symptom of the Hamilton Rating Scale for Depression (HRSD) scores based on the symptom severity and pretreatment electroencephalogram (EEG) that records the electrical signals of the brain.
“The machine learning model achieved C index scores, indicative of discriminative performance, of 0.8 or higher on 12 of 21 clinician-rated symptoms,” wrote the researchers in the study. ElecTreeScore produced the highest C index scores for predicting improvement for symptoms of weight loss, unreality and nihilism, and loss of insight. The team concluded that machine learning may be used to “identify independent associations of symptoms and EEG features to predict antidepressant-associated improvements in specific symptoms of depression.” As next steps, the researchers suggest prospectively validating the approach in clinical trials and settings.
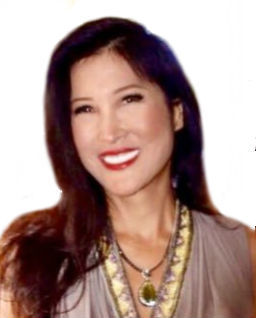
Cami Rosso writes about science, technology, innovation, and leadership.
psychology today